GroundCover™ Issue: 135 July - August
Author: Dr Gio Braidotti
Private and public sector research collaboration is fast-tracking breeders’ cumulative progress towards increasingly higher-performing crops.
The high-yielding, drought-tolerant AQUAmax® maize hybrids have been a remarkable success in the US, where they are grown on more than 3.6 million hectares. There are, however, three unusual facts about the hybrids that have important implications for the Australian industry.
First, AQUAmax® was bred by an Australian, Professor Mark Cooper, while he was working at DuPont Pioneer headquarters in Iowa, starting in 2000.
Second, Professor Cooper and his team of maize breeders did not use a traditional breeding program or GM technology to produce the hybrids. Instead, they developed prediction-based breeding methods.
These rely on huge datasets, mathematical relationships, genomic data and computer models – in short, computer intelligence – to predict which gene combinations are most likely to improve crop performance in relation to environmental conditions and farming practices.
And third, Professor Cooper has relocated from DuPont Pioneer back to academia, where he intends to accelerate adoption of prediction-based methods in the public sector while also training a new generation of breeders.
Professor Cooper has accepted the chair of Prediction-Based Crop Improvement at the Queensland Alliance for Agriculture and Food Innovation (QAAFI), based at the University of Queensland.
Australia an obvious choice
Professor Cooper says the University of Queensland was the obvious choice when it came to bridging public and private sector capabilities with the new breeding technology – a choice that DuPont Pioneer supports. “The University of Queensland is a ‘hot’ environment to do this work,” he says. “You don’t have to spend time explaining what prediction-based agriculture is. That means I can come in and work in a complementary role to teams already engaged in this space.”
It is where artificial intelligence is being trained to deliver ‘genomic prediction’ capability (see GroundCover™ issue 134, ‘Could AI deliver the next wheat breakthrough?’).
It is also where another GRDC-sponsored chair, Professor Graeme Hammer, led a team that developed the crop growth model called the Agricultural Production Systems sIMulator or APSIM (see GroundCover™ issue 88, ‘Computer power starts to build risk-management muscle’).
The aim of Professor Cooper’s new role is to create a win-win situation for the public and private sectors. Benefits for the private sector include closing the gap between how students are trained and how they are likely to fit into commercial breeding roles in the future.
Conversely, public breeding programs can overcome the need for large datasets and financial investment by implementing aspects of predictive breeding that have the highest pay-off.
“The new technology allows for more strategic, cost-effective investment in crop improvement in the public sector,” he says.
Professor Cooper’s first priority is proof-of-concept studies that target performance gains in wheat and sorghum using prediction-based methods. He will collaborate with QAAFI-based crop improvement teams led by Dr Lee Hickey on wheat and Dr David Jordan on sorghum.
Innovation cycle
While it is multinational breeding companies that have matured prediction-based breeding methods, Professor Cooper says that past partnerships with the University of Queensland were essential to the evolution of this technology.
In the 1990s, DuPont Pioneer jump-started its modelling capability via a research partnership with Professor Hammer and an APSIM licensing agreement. The company then built an internal R&D team that continued the partnership and developed parallel modelling capability more suited to an industrial breeding program.
In 2000, Professor Cooper transferred from the University of Queensland to the DuPont Pioneer side of the collaboration and, in 2011, the company released the first generation of AQUAmax® products.
“Work done through the collaboration was used in the development of a leading DuPont Pioneer brand,” Professor Cooper says.
“Now we want to complete the innovation cycle we started in the 1990s, with a return of expertise back to the university in what amounts to the creation of an almost open innovation system.”
How prediction breeding works
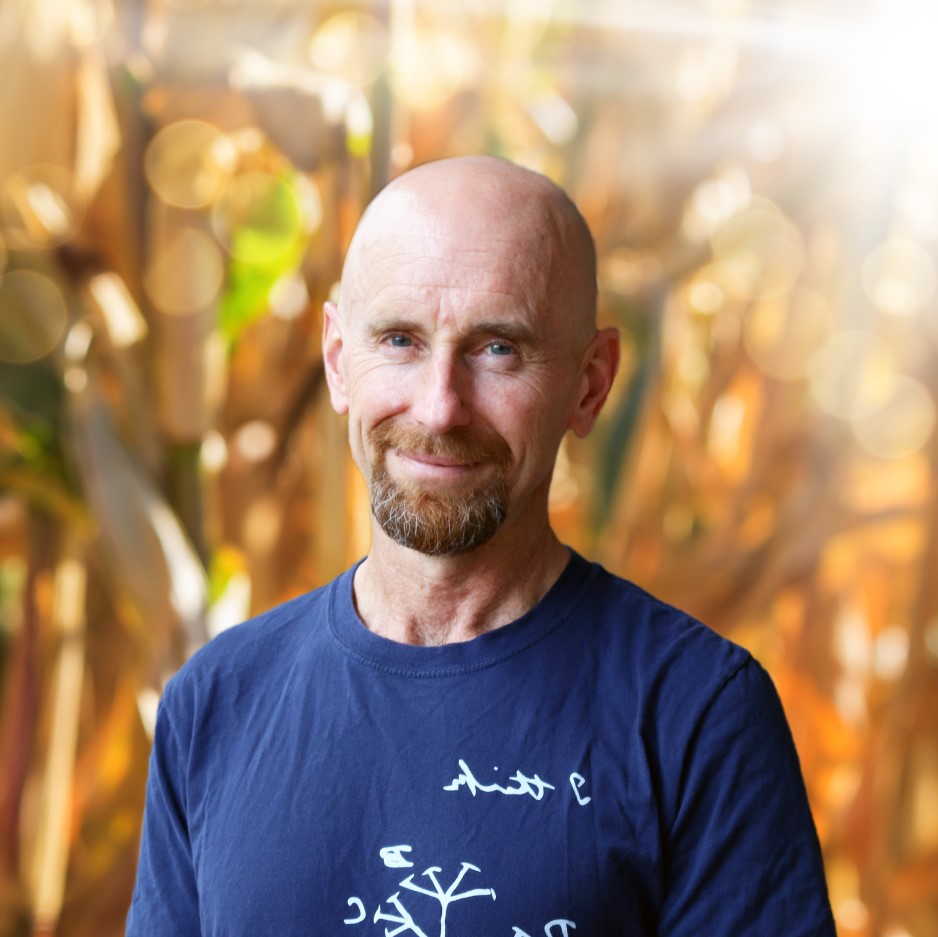
The prediction-based methods developed by Professor Cooper for DuPont Pioneer basically involve melding two different modelling capabilities.
First, there are quantitative genetics models. These rely on comparing the performance of genetically diverse plants in field trials to detect the DNA sequences that can account for high-performing traits.
While these models have some predictive capability when plants are grown under standard conditions, they notoriously struggle to predict how plants will respond to variation in the environment or to varied management practices.
However, starting in the 1990s researchers at the University of Queensland developed a different kind of computational tool – a crop growth model.
This software simulates physiological processes that are essential to growth, including processes that interact with the environment, such as the availability of light, water and soil nutrients.
As such, they do not deal with ‘genetic traits’, but rather work one level up in scale, with what Professor Cooper calls ‘intermediate traits’. Examples include photosynthesis in the leaves or nutrient and water uptake in the roots.
“Rather than trying to associate a gene with yield, we work with those clusters of genes that underlie the ‘intermediate traits’ – the same traits captured in crop growth models,” Professor Cooper explains.
The advantage is that the combined models can better predict how genes selected during a breeding program are likely to interact with seasonal weather variation and agronomy. As such, they can account for the all-important gene-by-environment-by-management effect (GxExM).
“Instead of being surprised at each turn of a breeding program, predictive modelling means we can ‘preview’ unique trait combinations and understand which increase crop performance,” he says. “This allows us to scale a breeding program even when it involves complex traits (such as drought tolerance).
The predictive software can subsequently even be used on-farm to predict crop performance and better manage risks.”
Professor Cooper says DuPont Pioneer is already in its third iteration of prediction-based breeding technology. “So when people ask us to demonstrate that this technology will work, industry has already answered that question and moved on. It has already decided this technology is the future.”